MRP is not junk if you actually do it right 📊 July 19, 2020
Or, why prominent media analysts should show their work
Welcome! I’m G. Elliott Morris, a data journalist and political analyst who mostly covers polls, elections, and political science. Happy Sunday! This is my weekly email where I write about news and politics using data and share links to what I’ve been reading and writing.
Thoughts? Drop me a line (or just respond to this email). If you like what you’re reading, tap the ❤️ below the title; it helps the post rank higher in Substack’s curation algorithm. If you want more content, I publish subscriber-only posts 1-2x a week.
Dear reader,
Newspapers, and in particular some prominent data journalists, are doing science wrong. They make sweeping claims about methods but often do not share their code. Some also have massive followings, online and off. When they speak about statistics and statistical methods, people listen. If they showed their work, or cooperated on analyses, we could be giving people the best information possible.
Why prominent media analysts should show their work
We can either be selfish or scientists about the insights we produce
Let me just echo everything my colleague Andrew Gelman said in this blog post about Nate Silver taking more shots at MRP (multilevel regression and poststratification) this week. Nate tweeted on Thursday that MRP produces predictions that aren’t as good as poll-aggregation. For the uninitiated, MRP is a method that takes raw polling data, learns about the relationships between demographic and geographic traits with attitudes and projects them back onto populations. It lets us turn national polls into state polls, for example—or even state polls into state polls!
But actually, what Nate said is “trying to impute how a state will vote based on its demographics & polls of voters in other states is only a mediocrely accurate method,” which isn’t really MRP at all, that’s just regression.
So we asked Nate for code, and he hasn’t shared it. This makes me think he’s just running MRP wrong. Or maybe he is and he knows something that none of us know. I’ll note that some analysts did MRP on Gary Langer’s ABC/WaPo polling in 2016 and actually beat the polling aggregators. It would be helpful f Nate could explain why that’s wrong.
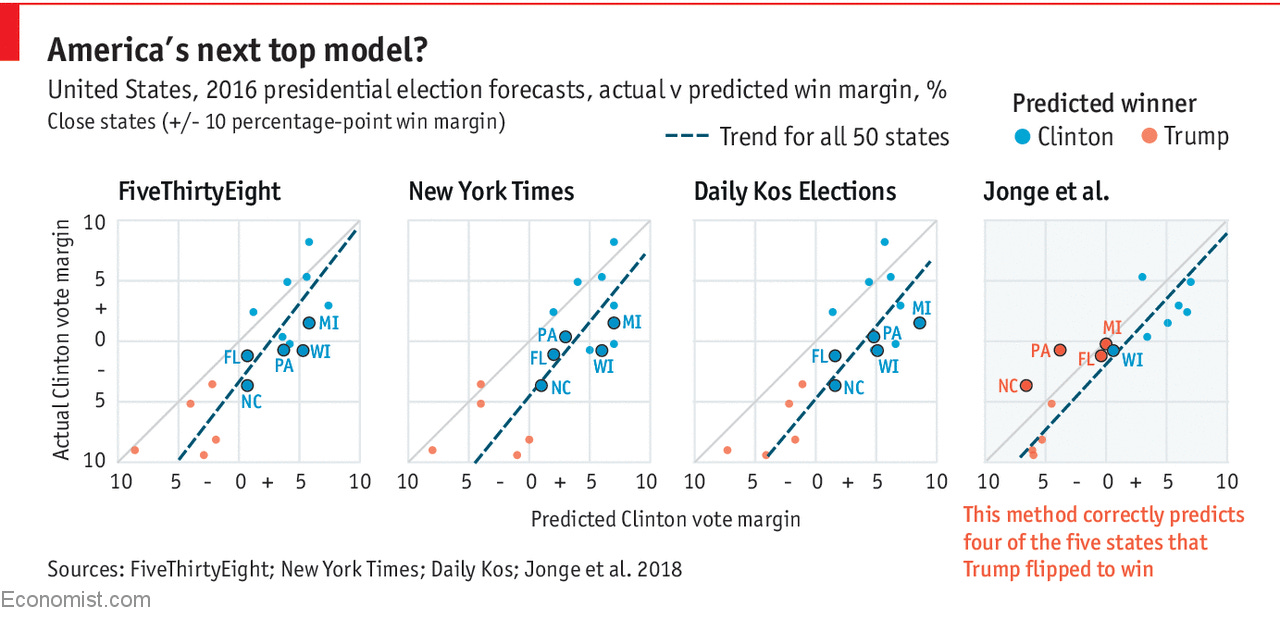
I don’t want to relitigate the MRP wars here, though. I do think it’s reasonable to take all the paths we can to better predictions—what Andrew has called “cooperation, not competition” (of methods). But instead, I just want to make a few points about data journalism and science—applying the “cooperation, not competition” philosophy to meta-learning between journalistic outfits.
Frankly, I think many data journalists are too siloed. I’ve experienced this among one or two of my peers, who are averse to sharing data with our leading competitors in certain scenarios, but mostly they do a good job and have improved recently. The Economist publishes a lot of the data and code for our data journalism now, and we’ve even released the whole enchilada for our 2020 presidential election forecast.
It is worth considering the motive for the siloing. I imagine that the major motivation for Nate not showing his MRP work, for example, is that he doesn’t want to give away his secret sauce. He has, of course, been pretty successful with this strategy so far. And he’s also a bit self-interested in defending the method that has made him rich and famous. But it’s not just Nate. Few analyses from the New York Times are ever made public, and The Washington Post’s code blog is less about proving findings and more about dev topics—though both have improved recently (Lenny Bronner at WaPo published their live election-night results-prediction model, and Nate Cohn shares some of their raw polling data on GitHub).
But siloing is not science. And though journalists aren’t explicitly in the business of teaching people about the world, or about teaching them the best methods of learning about the world, I would argue that data journalists are—and Nate certainly is. His book is recommended reading for courses in statistics and empirical journalism. I think data journalism in general fills a void for instructive, empirical—and objective—journalism. We should try to shore up that mission as much as possible.
Indeed, I think that the one big strength of data (or computational or empirical or whatever) journalism is that the work is verifiable. It’s also iterative. If we publish something with a coding error, people can go in and tell us and improve the reporting—improve what we’re learning about the world. But they can only do that if we share our work. And if you adopt open science as the default for data journalism the alternative looks all the worse. Why wouldn’t we want people to see our work and point out our errors? Or take our perfectly-fine code and add to it their own analyses?
To me, the choice between science and selfishness is clear. We should be as transparent as possible in the work we produce. That way the community can make it the best it can possibly be. Nobody should own any knowledge, and if they’re going to share it, they should be fair and transparent when doing so.
…And that’s why Nate should show his work for MRP. Because, if he’s actually doing it right or with the “best” data sources, it should roughly meet the performance of topline poll aggregation on average, and could even be quite a bit better. And if he’s not getting those results, we can figure out why.
Nate also tweeted yesterday that “when it comes to working with data, building models, etc that it helps to be a bit self-taught.” I agree (and I did teach myself most of the advanced stuff that I know about stats/programming) but I would also in response say that maybe if Nate were willing to learn from others a bit more, he would be getting the conversation about MRP right.
Be sure to read Andrew’s post; his has cat pictures.
Posts for subscribers
July 16: It’s really looking like 2008 again. The share of adults aligned with the Republicans has taken a sharp dip this summer
What I'm Reading and Working On
I’ve been reading the classics recently. V. O. Key, James Bryce, Warren Miller. I need a break, so it’s time to finish Lord of the Rings.
Nathan Kalmoe has a new book coming on how partisanship shaped violence before and during the Civil War. I liked his last book on mass ideology and am really looking forward to this one (when I can make the time).
Thanks for reading!
Thanks for reading. I’ll be back in your inbox next Sunday. In the meantime, follow me online or reach out via email if you’d like to engage. I’d love to hear from you!
If you want more content, I publish subscriber-only posts on Substack 1-3 times each week. Sign up today for $5/month (or $50/year) by clicking on the following button. Even if you don't want the extra posts, the funds go toward supporting the time spent writing this free, weekly letter. Your support makes this all possible!