Just how useful are the polls? 📊 September 13, 2020
Another newsletter on the true scale of uncertainty in public opinion polls
2020 has been one of the most eventful election years ever, yet 250 days have passed with little mark of the elapsed time. Maybe my middle school obsession with Doctor Who was a hint at a future to come? And this is my newsletter.
As always, I invite you to drop me a line (or just respond to this email). Please click/tap the ❤️ beside my name if you like what you’re reading; it’s our little trick to sway Substack’s curation algorithm in our favor. If you want more content, I publish subscriber-only posts 1-2x a week.
Dear reader,
There are just over seven weeks—fifty-one days, to be precise—until Election Day. There is one big question on my mind: how much can things change? That’s the question that we attempt to answer with election forecasting models. We combine data on the “fundamental” state of the race—stuff like the economy and presidential approval ratings have a good track record of projecting outcomes—to forecast a base scenario for the contest and then add polls on top of that. Which raises a question: how much are polls worth? I shared a few tweets about this on Twitter and will use this week’s newsletter to expand on them.
—Elliott
Just how useful are the polls?
Another newsletter on the true scale of uncertainty in public opinion polls
So we got into a discussion about margins of error and the value of polling data (again) on Twitter today. Here are the three main tweets, though there is some other related back-and-forth in the threads:




OK, here’s the rub.
As I’ve written about before, polls are only rough indicators of election outcomes. That’s due to two primary (and inherent) shortfalls in the method of surveying the public.
The first is that you can’t survey everyone! And that creates random error in how well your poll will match the target population. Maybe you don’t get enough people of color, seniors or rich people. This is the type of error that a poll’s traditional “margin of error” tries to capture. We will call this the “margin of sampling error.”
But there is a lot of extra error in polls besides the traditional uncertainty from random sampling error alone. That’s because polls today have a lot of trouble randomly sampling the population in demographically and politically representative ways. Not everyone has a landline or cell phone, for example; and the people who don’t are different than those that do. This is a weakness for online polls too. In other words, randomly sampling phone numbers or emails (or panel respondents, etc) from those populations of people who you can contact might not match the actual population of adults who you’re trying to reach.
Then, there’s error from who is likely to actually (a) answer your poll and (b) finish the survey all the way through (what pollsters call “completes”). We know that college-degree-holders are more likely to talk to pollster, for example, which further biases polls toward the opinions of college-educated people. This is a type of “non-response” error. Another important type is “differential partisan non-response,” in which a news event or other phenomenon might cause members of one party to refuse pollsters’ calls or questions at higher rates than members of the other party.
All of this potential error means that pollsters have to come up with ways to adjust their data to make it representative—both demographically and, ideally, politically. The most common technique is to give more weight to certain respondents to ensure that the balance of white and black, rich and poor, educated and uneducated adults matches the breakdown of those attributes according to the Census.
But this weighting doesn’t get us out of the woods! All of these adjustments can decrease the precision of pollsters’ estimates. Good firms will account for this loss of precision, but many only report the uncertainty from the margin of sampling error, which understates the true variance for a poll.
We should discuss one other major consideration, particularly for pre-election polls. When trying to estimate attitudes among the voting electorate, pollsters run into the age-old problem that the precise demographic and political characteristics of people who actually turn out cannot be known in advance. We have no Census data on how many Boomers and Millennials, Democrats and Republicans will vote on election day because that target population doesn’t exist yet!
So pollsters make guesses. That’s where we see so-called “likely voter filters” come in. Here, pollsters will filter their samples to only include people who say they’re likely to vote, or they might modify the weights for each person based on their predicted likelihood of turning out. This is all a bit complicated, but the takeaway is that making guesses about who is going to vote on election day (which every pre-election poll does in one way or another) increases potential error even more.
In the end, this means that polls have two major categories of error: sampling error, the traditional uncertainty quantified by the margin of error, and non-sampling error, which pollsters often don’t report or account for. This is why it’s a good rough guide to assume that the margin of error for an election poll is closer to twice as much as the one the average pollster reports.
As I said in my tweet, this is why it’s good to train your brain to see polls as fuzzy estimates, not the word of god.
…
When I tweeted about the true scale of error in pre-election polls this morning, I also got asked: “then why use them in models?” I have blogged about this before, but let me briefly answer by saying that building a model of elections allows us to accurately account for the full range of uncertainty in the data and formalize how much polls should influence our beliefs given said error. In fact, in some ways a model is the only way to do this—unless you’re steeped in the ins and outs of survey research, you likely don’t know about how uncertain polls really are. Building a model lets us convey that fact, not obscure it.
Posts for subscribers
September 11: This is exactly how covid-19 costs Trump voters. Voters punish the president when people around them die under his watch
September 9: A Labor Day update to the presidential campaign. The horses are rounding the second bend
What I'm Reading and Working On
With the election season coming to a head, I’m spending more and more time looking at polling data and less time reading—so no book recommendations this week. Though I did think this Atlantic article on how campaigns are (or, rather, are not) targeting Latinos was insightful.
Next week’s articles will be about campaign finance and rural voters.
Thanks for reading!
Thanks for reading. I’ll be back in your inbox next Sunday. In the meantime, follow me online or reach out via email if you’d like to engage. I’d love to hear from you.
If you want more content, I publish subscriber-only posts on Substack 1-3 times each week. Sign up today for $5/month (or $50/year) by clicking on the following button. Even if you don't want the extra posts, the funds go toward supporting the time spent writing this free, weekly letter. Your support makes this all possible!
Photo contest
This week’s winner is Matthew from North East England. Joe, Matthew’s human, says he is “excited for some dreamies.”
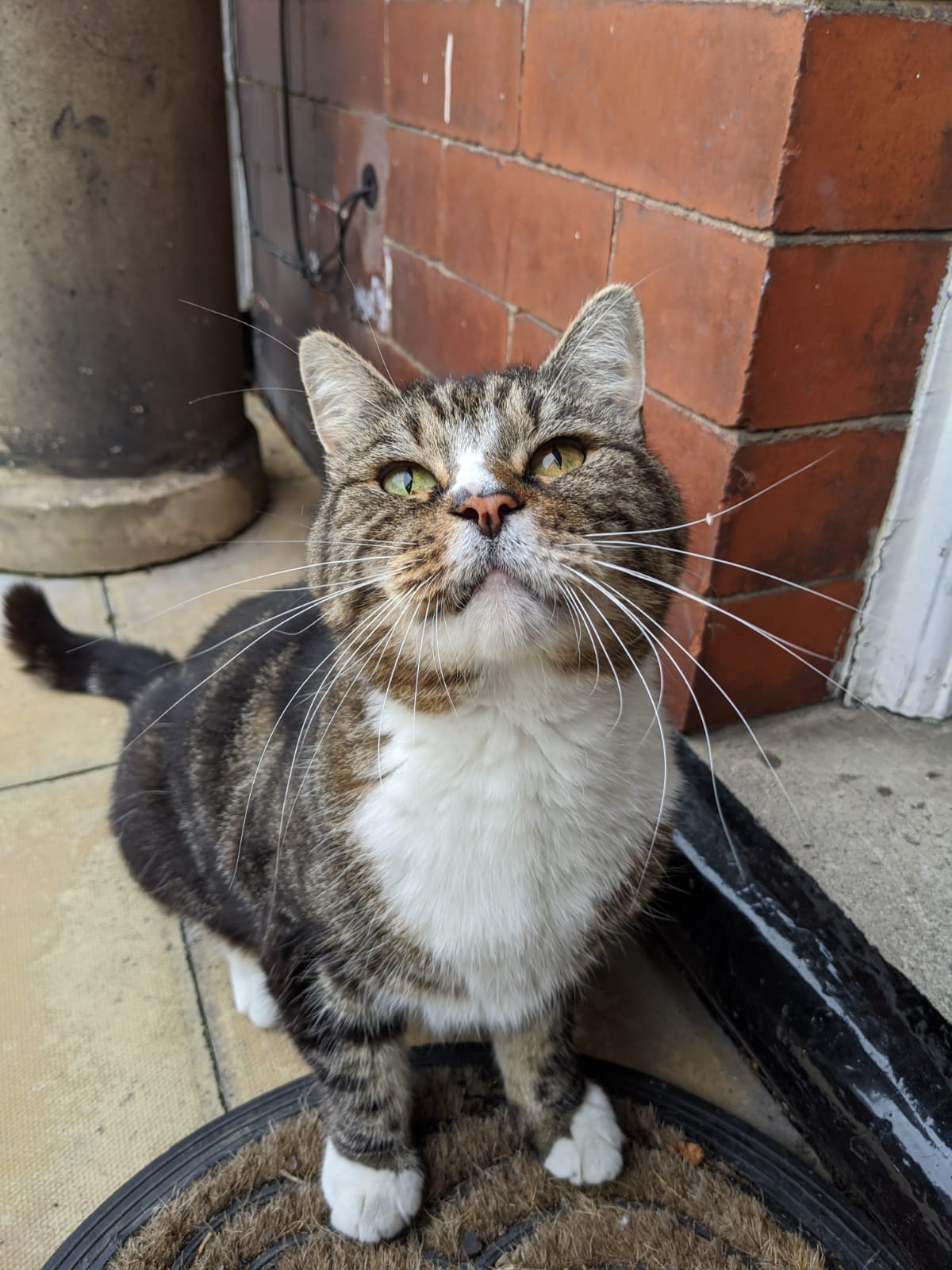
For next week’s contest, send in a photo of your pet(s) to elliott@gelliottmorris.com!