Brief thoughts about The Election Forecast Wars
What can we learn when models (and modelers) disagree?
Friends,
David Byler, a data analyst and opinion writer at the Washington Post, published a new column today about “The election forecast wars” that I have thoughts about. Let me also say up top that I think David is a good commentator and an astute election forecaster, and my thoughts are less about him and more about extracting signals from the noise of intellectual disagreements.
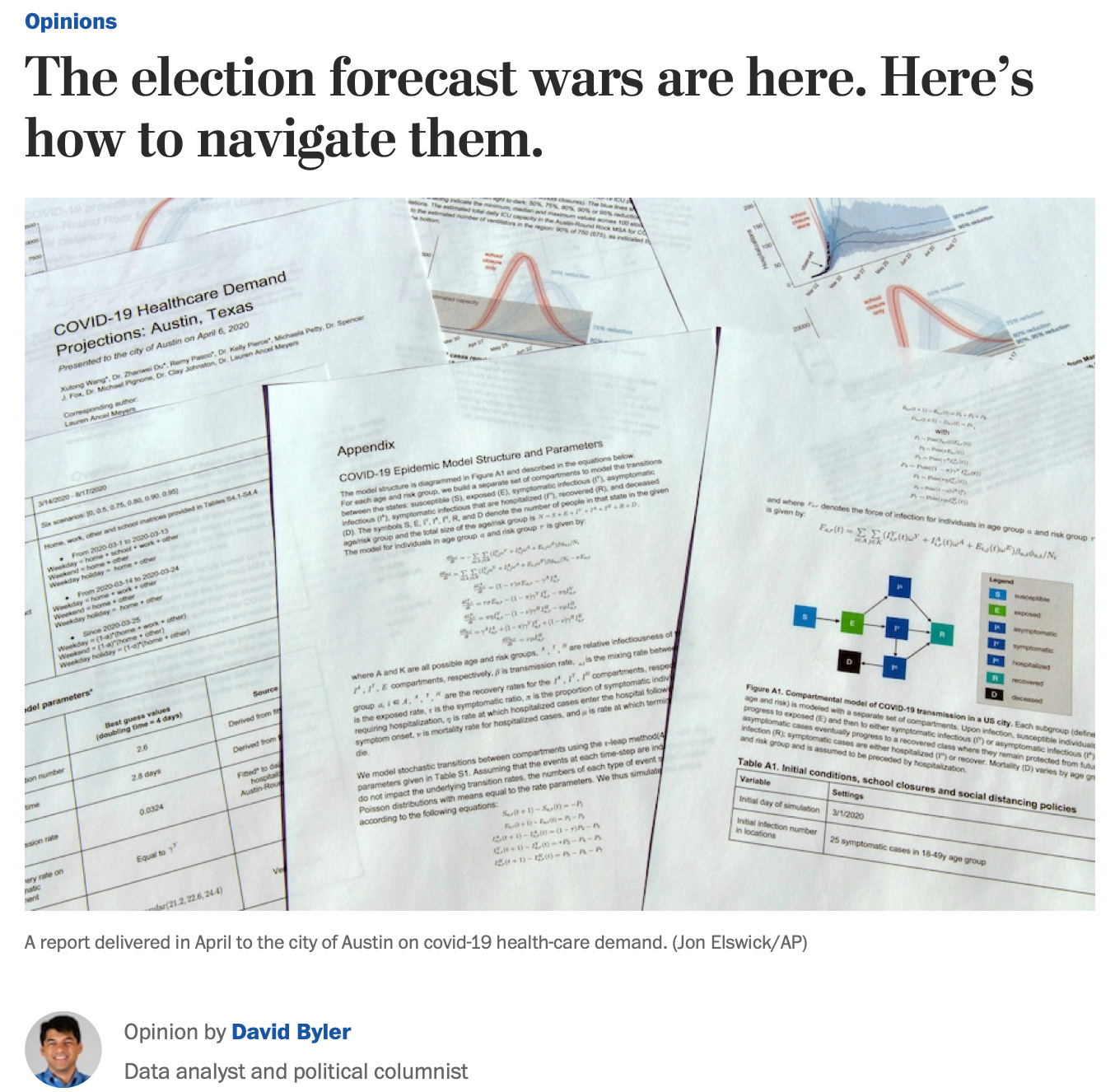
My biggest objection to the piece is the headline: “The election forecast wars are here. Here’s how to navigate them.” I think this is an obnoxious way to make the otherwise intellectual (well, typically intellectual, but not always) disagreements that Nate Silver and I have been having publicly into some sexy tabloid-esque story that is focused on conflict, not on learning. It’s also a bit annoying that piece also doesn’t really tell me how to navigate election forecasts or the wars, but rather presents three “rules” that are helpful when reading forecasts. Those rules are helpful, but I feel misled! I’m sure that this isn’t all David’s fault (headline writers are people that exist) but it still irks me.
Anyway, here are Byler’s rules:
The first rule of model reading: Don’t check your brain at the door. (Basically, models aren’t perfect and you should screen them with common sense.)
The second rule: Watch out for events that blow up old patterns. (Models built on inflexible quantifications of history can be overfitted and unhelpful.)
Which brings me to the third rule: Take good models seriously. (Good models can be useful.)
I think the rules are good rules for evaluating forecasts, but I don’t think we’re really getting to the crux of how to navigate The Election Forecast Wars (TM). What are the disagreements? What can we learn from them? Let me give this a shot:
1. Modern elections are fundamentally different from the old ones
This is the first point of disagreement that Nate and I have had. It shows up in multiple parts of our modeling.
First, whereas he thinks it is appropriate to compare the relationship between economic growth and election outcomes from the 1880s to the modern day, I think that such an assertion is pretty odd. You can think of a lot of reasons why this might be the case. The media has certainly changed the way that the conversation over the economy is framed and debated, for example. Polarization also means that voters don’t care as much about the economy as they used to (something that Nate’s model ignores).
2. The way we treat polls should also change over time
The hypothesis that polarization has fundamentally changed the electoral landscape also shows up in how much polls can swing from day to day, and also how high or low they can go for any given candidate (what we call their ceiling and floor). In elections since 2000, the standard deviation of polling averages has been less than half of what it was in the elections beforehand. A model should know this, and Nate’s doesn’t.
The lower variance in recent elections might not be entirely due to polarization, to be sure. We also have more polls to put in our averages now, which should have the effect on average of making averages that are less jumpy. But we’re not concerned with small day-to-day movements, but with large weekly or monthly changes in the horse race. And those have evidently gotten less frequent over time.
3. Presidential approval is a helpful factor in predicting elections
In recent days, Nate has also spit on models that include presidential approval as a predictor for the November horse-race outcome. He says that you shouldn’t do this because approval “is the dependent variable” but doesn’t really add more to the objection. I think this ignores a few things.
First, that approval rating provides information about the fundamental state of the electoral environment that might not be captured by trial-heat polls. Particularly before people are tuning into the race—eg before the conventions—presidential approval ratings provide a good baseline measure of how people feel about the incumbent party, and how the polls might move over the course of the election cycle.
Which brings me to the second factor: The information contained in approval ratings is even more relevant when an incumbent president is on the ballot. It provides a very predictive baseline reading of the November outcome, especially early in the election cycle. Approval ratings can also be used to hint at how undecided voters might feel about the candidates, ie their underlying or fundamental predisposition toward the party or president in power.
Finally, there’s a point to be made (not worshipped) about prediction. An average of presidential approval rating polls is one of the most predictive indicators of the election result (again, especially early on in the cycle). In fact, it’s much better at anticipating the vote than an index of economic growth. So, if you have a good theoretical motivation for including it in your model, you should. (NB: This helps explain why Nate is always saying that the “fundamentals” are actually pretty bad predictors of the outcome. It’s because he’s excluding the most predictive one!)
I think we now have a good understanding of why we should treat approval ratings as a “fundamental” indicator that should be included alongside economic growth in any reasonable model of the election. In my opinion, Nate is leaving this information off the table without good reason.
4. The only way to test a model is to make predictions before November
One of the bigger disagreements I have had with Nate concerns what we can learn about how good a model is, and how we can learn it. I discussed this in a post a few weeks back and don’t want to rehash all of it.
The thrust of my argument is that testing a reasonably-specified model on data that it has not seen before is a pretty good measurement of its quality, even if it won’t perfectly predict how the model will do in the next election. Nate disagrees; he says the only way to evaluate a model is if it has a track record making predictions of election outcomes before November. (NB: Nate uses this argument to justify why people should trust his 2020 model more than other models, conveniently leaving out the fact that his 2020 model has a whole lot of new variables that he didn’t test on the 2016 version!)
So there are some brief thoughts about our disagreements. This is by no means everything we disagree on, but it’s a good roundup of the broader themes and the ones I think most people will learn from. I’m sure we’ll revisit the subject over the coming months.
In the meantime, here are some related articles:
And don’t forget that I publish subscribers-only content once-to-twice a week!